1 SALESFORSALESFORCE FUTURESCE FUTURES ANTICIPATING PERSONAL AGENTS: FEASIBILITY REMEMBERING For an autonomous agent to be useful, it needs to have memory: the capability to store and retrieve information. Memory in AI is not a single entity, but rather a collection of layers. One layer is the ability for the personal AI to store and retrieve the prompts that the user initiates across multiple sessions. We refer to the ability for a personal AI to “stay” with a user over a longer time horizon (i.e. across multiple interactions) as persistence. The second layer is what’s already trained into the LLM and how the relationships are built between the pieces of data that inform the training. There are limits to this layer such as the cutoff dates for the training data. The third layer of memory is the ability of personal AI to retrieve data (both structured and unstructured) from other data stores by using methods such as RAG (retrieval augmented generation). Currently, context is provided by the user to the LLM, but utility is limited by the LLM’s inability to store the information provided (context length), and by the accuracy with which the user states their preferences. The user’s actual context may also include data the agent has no access to, thereby increasing the likelihood of “off” responses. Conversely, an agent might one day have access to the user’s entire recorded history, which would seemingly offer unlimited context (not to mention near unlimited data storage). But even if that’s the case, how does the agent know which parts of a user’s history are most important, or which parts are more sensitive? The challenges with memory and meaning for LLMs echo the challenges of understanding how humans assign meaning to memory. ISSUE 1: PERSONAL AI | 10
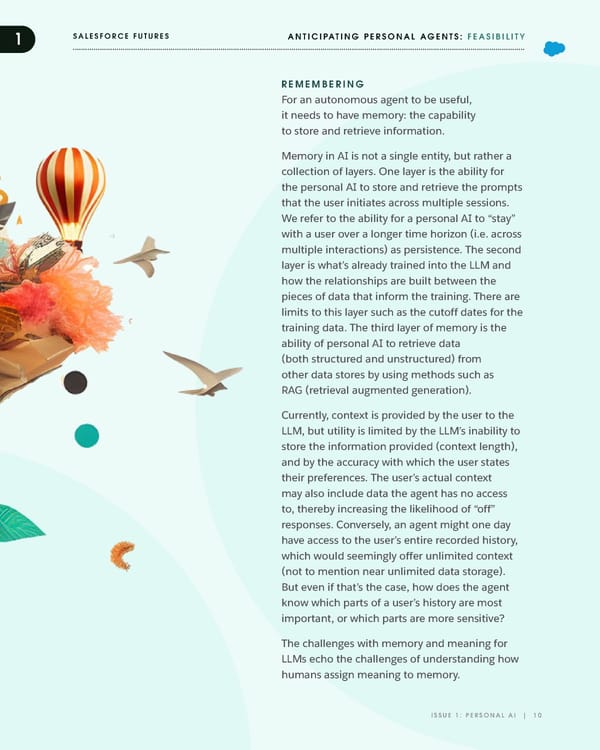